Chapter 5 Joint Distributions
5.1 Joint Densities & Independence
Goal: Look at pairs of random variables (X, Y);
result of experiment will give pair of values (one value for each)
ex:
Roll 2 dice; X = value on 1st die, Y = value on 2nd. Then the set of
possible results of the experiment are the pairs of values
{ (1,1), (1,2), (1,3), ..., (1,6), (2,1), ....., (6,6) }
Note: we'll consider only the case where the random variables
are discrete (not continuous)
Def: Let X, Y be discrete r.v.’s. Then their joint probability
density function is defined to be
f(x,y) = P( X = x and Y = y )
-
f(x,y) gives the probability that the outcome of the experiment will be
the pair of values (x,y)
-
can represent the joint density function as a table
ex:
Plants are grown in a greenhouse; suppose the number of stems and number
of blooms on each plant varies, with the number of stems being 1, 2, or
3 and the number of blooms being 0, 1, or 2.
Let X = # stems on a randomly selected plant: then possible values
are x = 1, 2, 3
Let Y = # blooms on a randomly selected plant: then possible values
are y = 0, 1, 2
Suppose that the joint density function of X and Y is given by the following
table:
What’s the probability a randomly selected plant will have 2 stems and
1 bloom?
We want P(X=2 and Y=1) = f(2,1)
= .25
What’s the probability a randomly selected plant will have more stems
than blooms?
We want P(X > Y); thus need to consider all
the pairs (x,y) where x > y, i.e.,
P(X > Y) = P(X=1 and Y=0) + P(X=2 and Y=0)
+ P(X=2 and Y=1) + P(X=3 and Y=0)
+ P(X=3 and Y=1) + P(X=3 and Y=2)
= f(1,0) + f(2,0) + f(2,1) + f(3,0) + f(3,1) + f(3,2)
= .22 + .09 + .25 + .01 + .07 + .09
= .73
What’s the probability a randomly selected plant will exactly 1 bloom?
We want P(Y = 1); thus need to consider all
the pairs (x,y) where y = 1, i.e.,
P(Y = 1) = P(X=1 and Y=1) + P(X=2 and Y=1)
+ P(X=3 and Y=1)
= f(1,1) + f(2,1) + f(3,1) = .12
+ .25 + .07 = .44
(Note that this just amounts to summing the entries in a particular column)
Properties
Let f(x,y) be the joint density function of discrete random variables
X and Y. Then
-
f(x,y) >= 0 for all x, y
-
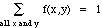
Can consider X or Y alone:
Def: The marginal density for X is defined as
fX(x) = P(X = x)
= 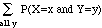
i.e.,
The marginal density for Y is similarly defined as
fY(y) = P(Y = y)
i.e.,
-
thus the marginal densities are obtained by summing either the rows or
the columns of the table for the joint density function
-
called marginal densities because the natural place to write them is in
the margins of the joint density table (see next example)
ex:
Consider the plant example above; find the marginal densities for X
and Y
X: fX(x) = P(X = x), so
fX(1) = P(X = 1) = P(X=1 and Y=0)
+ P(X=1 and Y=1) + P(X=1 and Y=2)
= f(1,0) + f(1,1) + f(1,2) = .22
+ .12 + 0 = .34
fX(2) = f(2,0) + f(2,1) + f(2,2)
= .09 + .25 + .15 = .49
fX(3) = f(3,0) + f(3,1) + f(3,2)
= .01 + .07 + .09 = .17
Notice that we're just summing each row of the table!
Y: fY(y) = P(Y = y), so
fY(0) = P(Y = 0) = P(X=1 and Y=0)
+ P(X=2 and Y=0) + P(X=3 and Y=0)
= f(1,0) + f(2,0) + f(3,0) = .22
+ .09 + .01 = .32
fY(1) = f(1,1) + f(2,1) + f(3,1)
= .12 + .25 + .07 = .44
fY(2) = f(1,2) + f(2,2) + f(3,2)
= 0 + .15 + .09 = .24
Just summing each column of the table!
These can be conveniently written right on the original table, in the
margins!
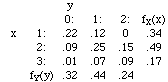
Independent Random Variables
Q: do the values for X and Y depend on one another, i.e., does
the value obtained for X influence in any way the value we get for Y? In
other words, is the event X = x independent of the event
Y = y ?
Recall:
two events A, B were defined to be independent iff
P(A
B)
= P(A) P(B)
So, letting A be the event that X = x and B be the event that Y = y, we
want to see if A and B are independent:
is
P(A
B)
= P(A) P(B)
i.e., is
P(X=x and Y=y) = P(X=x) * P(Y=y)
i.e., want to see if
f(x,y) = fX(x) * fY(y)
for all values of x and y
We use the above condition as our definition:
Def: discrete r.v.’s X and Y are independent
iff the joint density is the product of the marginal densities, i.e., iff
f(x,y) = fX(x) * fY(y)
for all values of x and y
-
random variables X and Y are independent if the value obtained for X doesn't
influence the value obtained for Y, and vice-versa
ex:
In the above plant example, the number of stems X and the number of
blooms Y are not independent. This follows because the joint density isn't
equal to the product of the marginal densities for all values of x and
y. For example, for x = 2 and y = 0,
but
fX(2) * fY(1) = (.49) (.32)
= .1568 = .16 (to 2 decimal places)
ex:
Roll 2 dice; let X = value on first die, Y = value on second
die. Then X and Y are clearly independent (the value on the first die can't
influence the value that appears on the second), and we can use this to
find the joint density function from the marginal densities using
f(x,y) = fX(x) * fY(y).
Since fX(x) = 1/6 for x = 1, 2,..., 6
and fY(y) = 1/6 for y = 1, 2,..., 6,
we get f(x,y) = 1/36 x, y
= 1, 2,..., 6.
But this is exactly what we'd expect: for example, f(3,5) = probability
that we get a 3 on the first die and a 5 on the second one, which equals
1/6 times 1/6! Thus we expect f(x,y) = 1/36 for all x, y.
ex:
Roll 2 dice; let X = value on first die, Y = sum of values
on the two dice. Then X and Y are not independent; the value on
the first die definitely influences the value that the sum can take on.
To show that the definition isn't satisfied, we need to find a pair of
values for x and y for which f(x,y) = fX(x)
* fY(y) doesn't hold.
Look at the case x = 1 and y = 12, i.e.,
the case where the value on the first die is 1 and the sum of the values
on the two dice is 12.
But this clearly can't ever happen: if the value on the first die is
1, the sum could be at most 7. Thus the value of the joint probability
function is 0:
f(1,12) = 0 ( P(X=1 and Y=12)
= 0 ).
Now look at the marginal density functions.
fX(1) = 1/6; the probability that we get a 1
on the first die (ignoring the value of the sum) is 1/6.
fY(12) = 1/36; the probability that the sum
of the two dice is 12 is 1/36, since the only way to get a 12 is to get
a 6 on each die, giving 1/6*1/6 = 1/36 for the probability.
Thus
fX(1) * fY(12) = 1/6 * 1/36
= 1/216
But thus
f(1,12) is not equal to fX(1) * fY(12),
so we've shown formally that X and Y aren't independent.
Previous section Next
section